Serverless Computing or FaaS is the best way to consume cloud computing. In this model, the responsibility for provisioning, maintaining, and patching servers is shifted from the customer to cloud providers. Which allows developers to focus on building new features and innovating, and pay only for the compute time that they consume.
In the last 7 months, we started using Lambda based functions heavily in production. It allowed us to scale quickly and brought agility to our development activities.
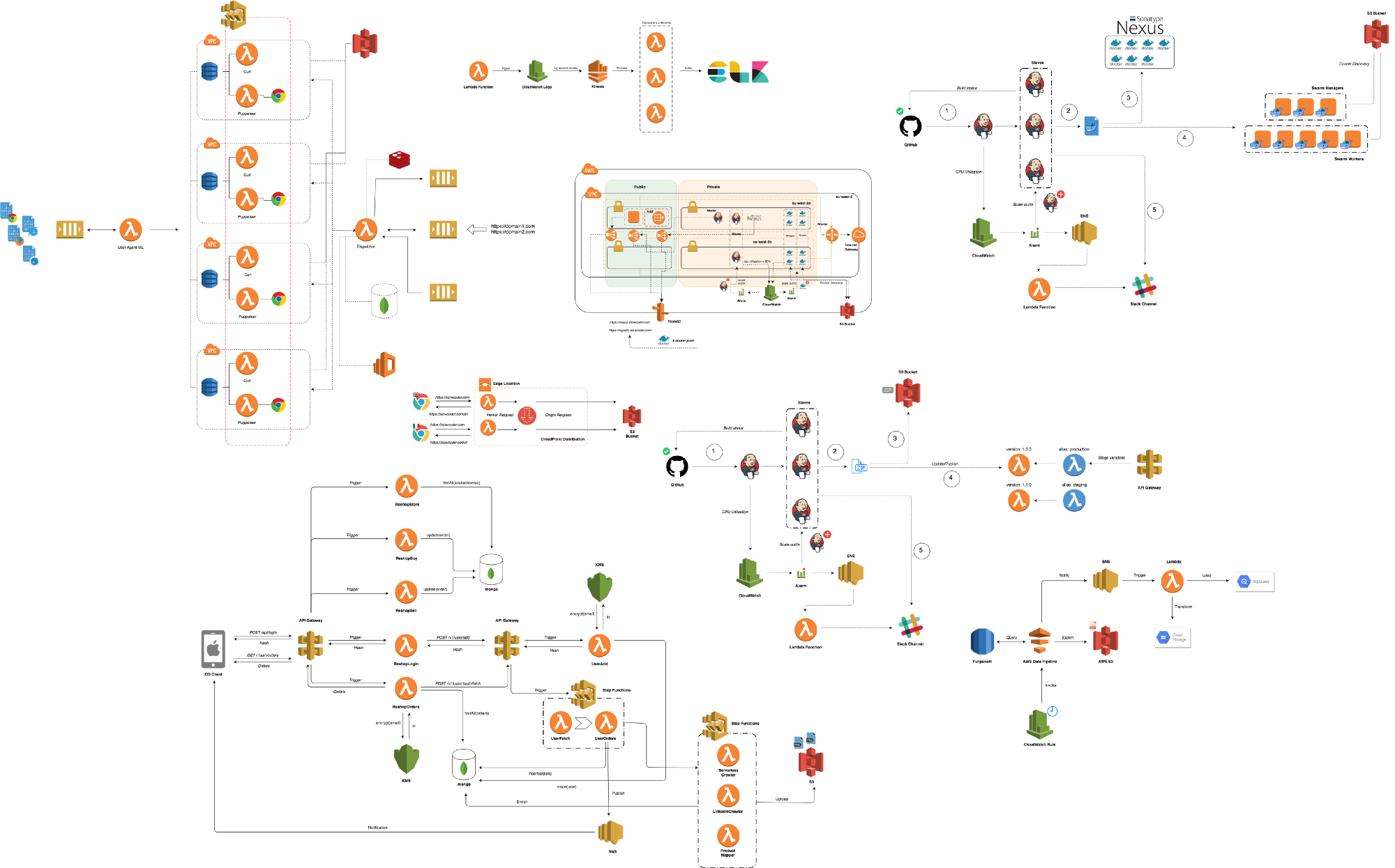
We were serving +80M Lambda invocations per day across multiple AWS regions with an unpleasant surprise in the form of a significant bill.
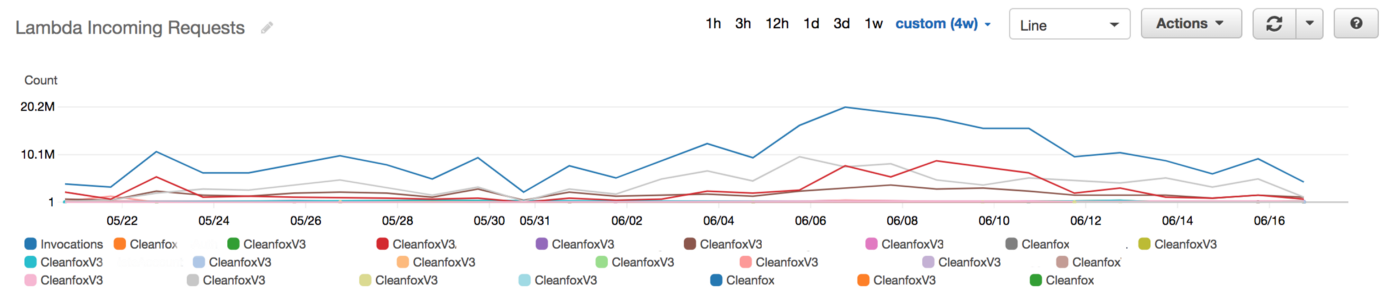
It was very easy and cheap to build a Lambda based applications that we forgot to estimate and optimize the Lambda costs earlier during development phase, so once we start running heavy workloads in production, the cost become significant and we spent thousands of dollars daily
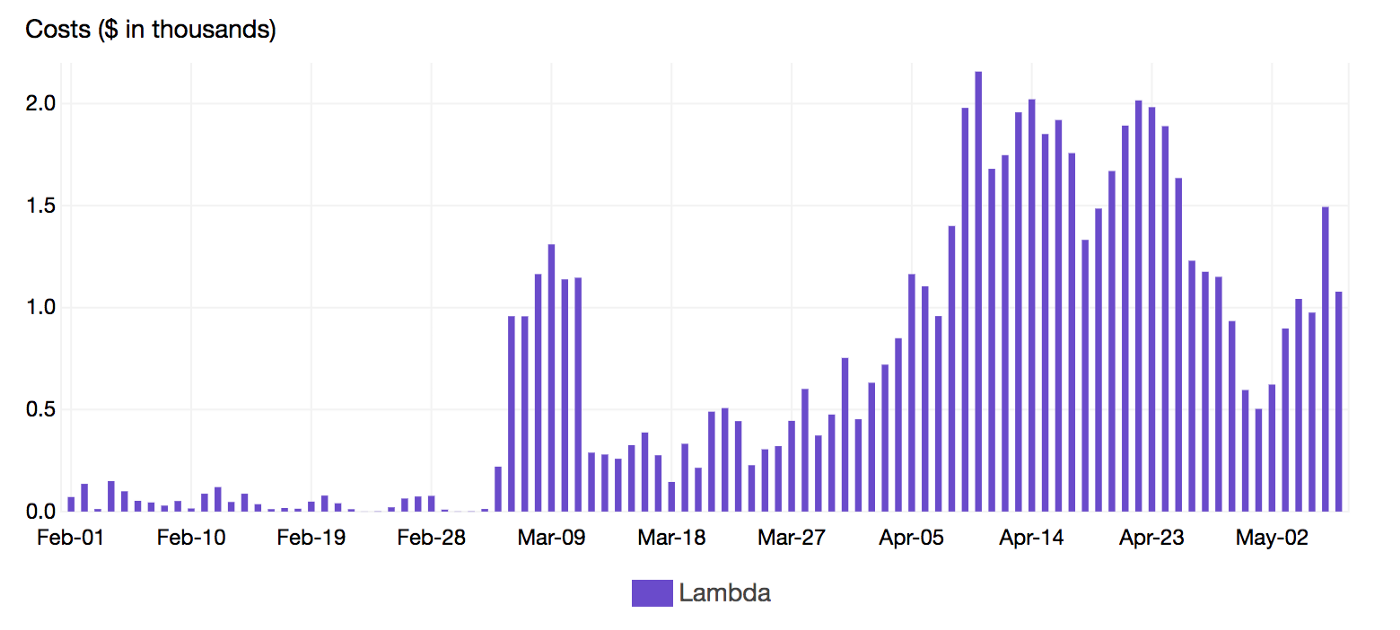
To keep Lambda cost under control, understanding its behavior was critical. Lambda pricing model is based on the following factors:
- Number of executions.
- Duration, rounded to the nearest 100ms.
- Memory allocated to the function.
- Data transfer (out to the internet, inter-region and intra-region).
In order to reduce AWS Lambda costs, we monitored Lambda memory usage and execution time based on logs stored in CloudWatch.

We’ve updated our previous centralized logging platform to extract relevant metrics (Duration, Billed Duration and Memory Size) from “REPORT” log entry reported through CloudWatch and store them into InfluxDB. You can check out the following link for a step-by-step guide on how to setup the following workflow:
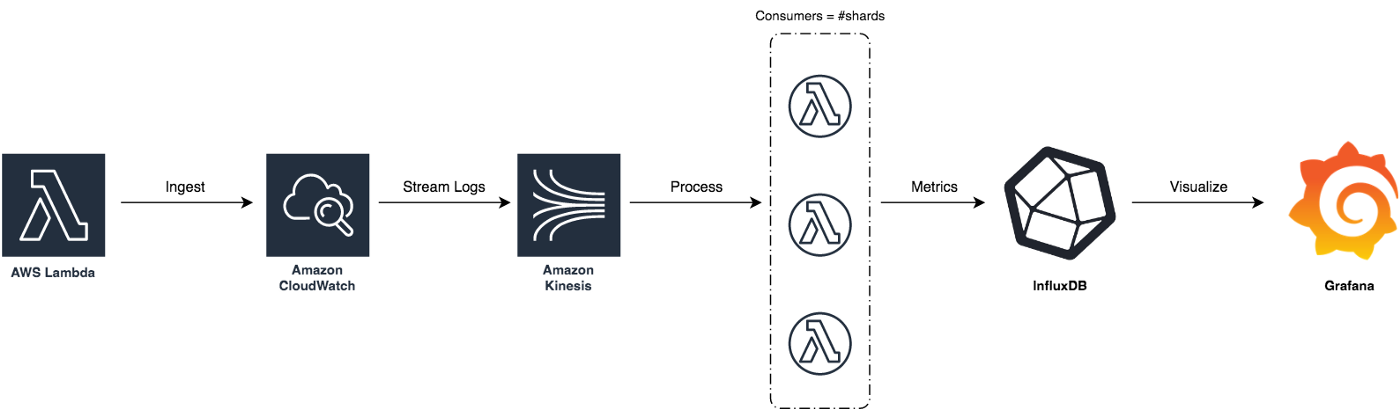
Next, we created dynamic visualizations on Grafana based on metrics available in the timeseries database and we were able to monitor in near real-time Lambda runtime usage. A graphical representation of the metrics for Lambda functions is shown below:
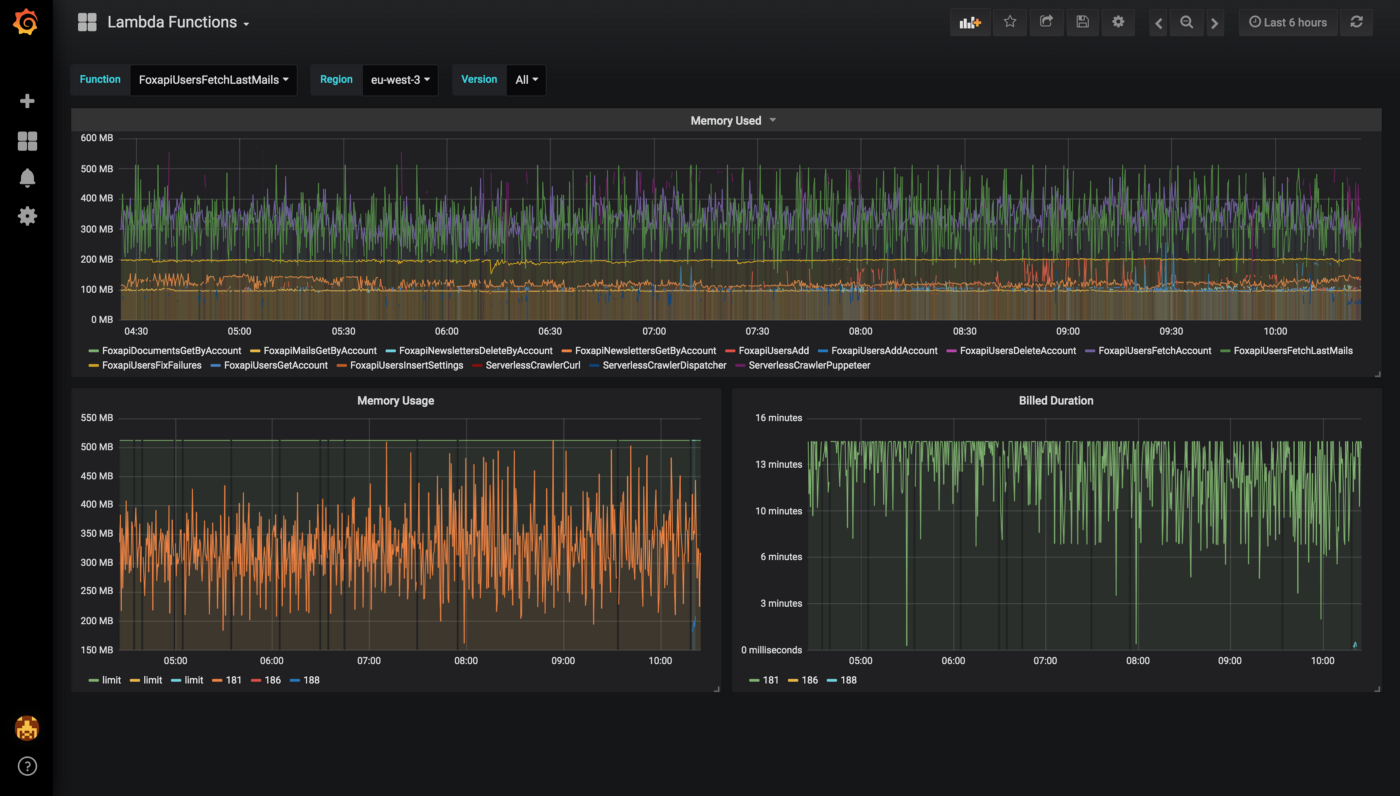
You can also use CloudWatch Logs Insights to issue ad-hoc queries to analyse statistics from recents invocations of your Lambda functions:
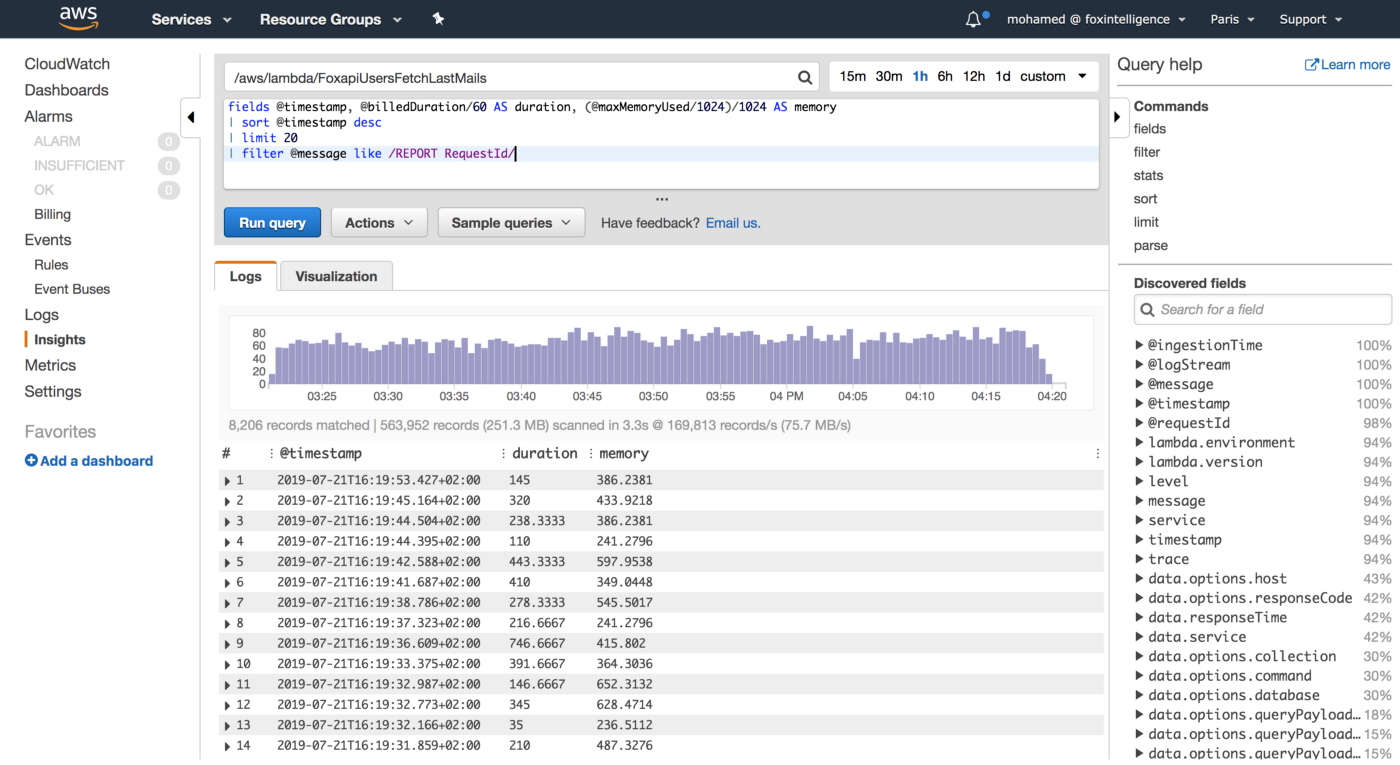
We leveraged these metrics to set Slack notifications when memory allocation is either too low (risk of failure) or too high (risk of over-paying) and to identify the billed duration, memory usage for the ten most expensive Lambda functions. When performing heuristic analysis of Lambda logs, we gain insights into the right sizing of each Lambda function deployed in our AWS account and we avoided excessive over-allocation of memory. Hence, significantly reduced the Lambda’s cost.
Memory allocation can make a big difference in your Lambda function cost. Too much allocated memory and you’ll overpay. Too little and your function will be at risk of failing. Therefore, you want to keep a healthy balance when it comes to memory allocation.
To gather more insights and uncover hidden costs, we had to identify the most expensive functions. Thats where Lambda Tags comes into the play. We leveraged those metadata to breakdown the cost per Stack (project):
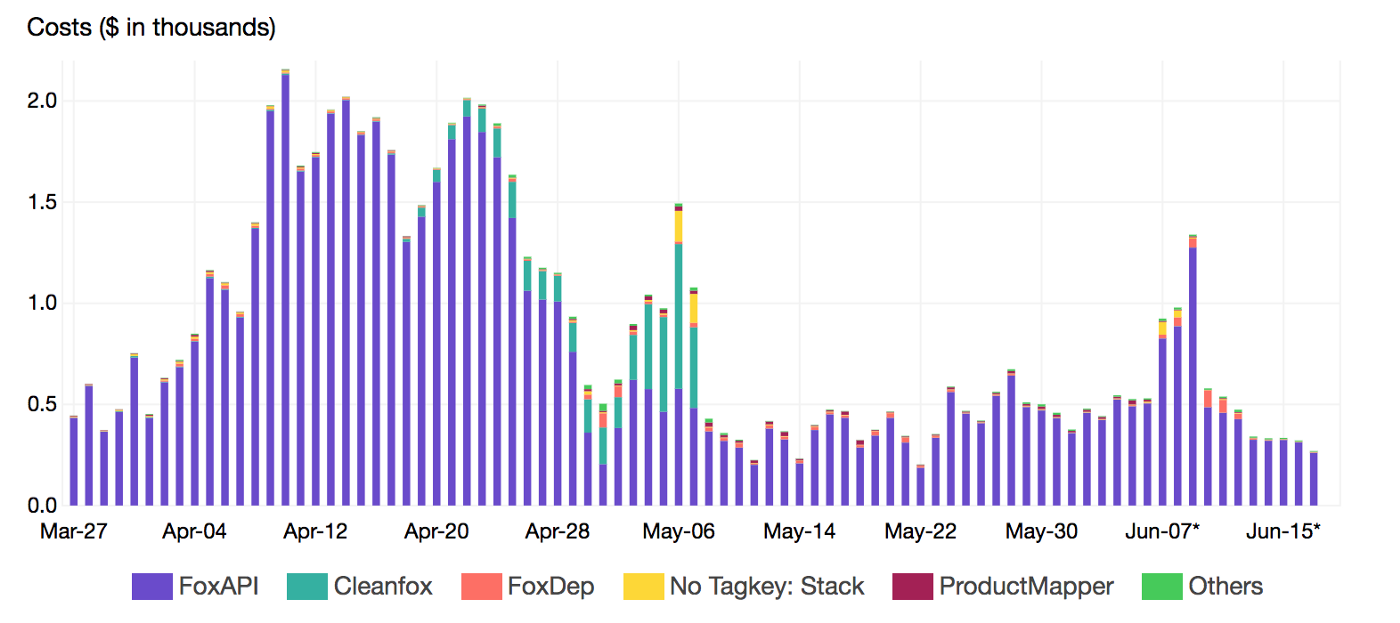
By reducing the invocation frequency (control concurrency with SQS), we reduced the cost up to 99% and CO2 emissions footprint of our B2C app Cleanfox 🚀💰
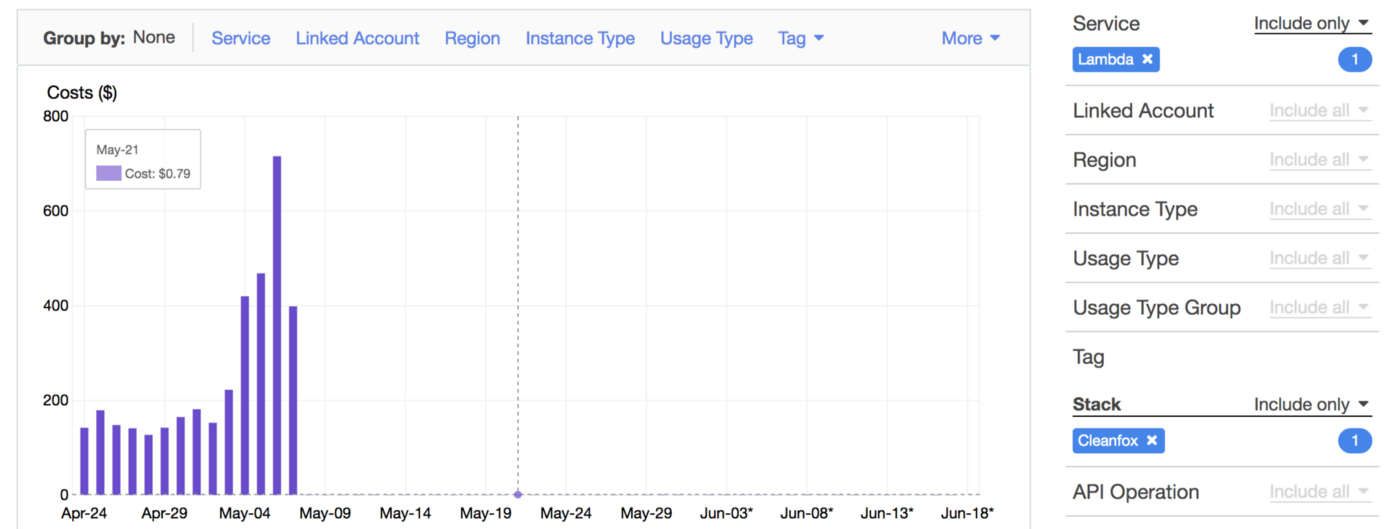
At a deeper level, we also breakdown the cost by Lambda function name using a secondary tag which is Function tag:
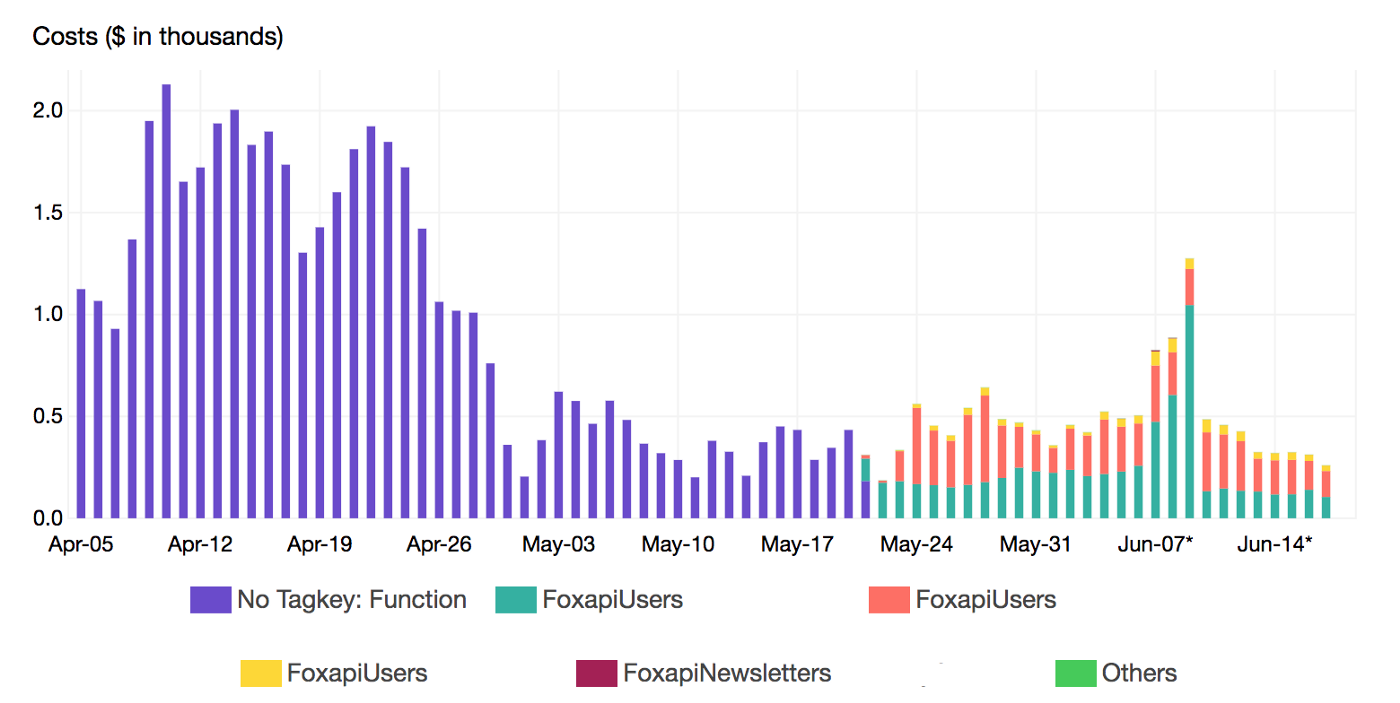
Once the target functions were identified, we reviewed the execution flow and applied some optimisation in our code to shorten the running time and resources needed (Memory and CPU)
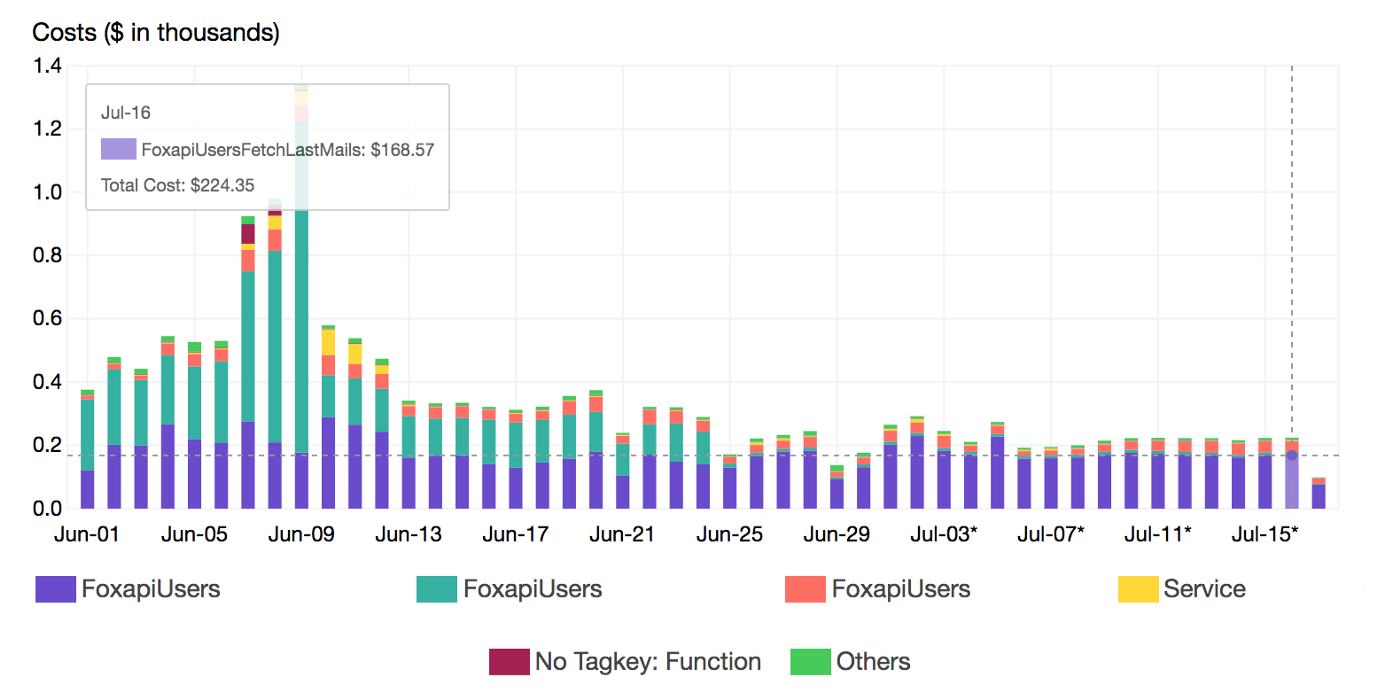
By continuously monitoring increases in spend, we end up building scalable, secure and resilient Lambda based solutions while maintaining maximum cost-effectiveness. Also, we are now configuring Lambda runtime parameters appropriately at the sandbox stage and we’re evaluating alternative services like Spot Instances & Batch Jobs to run heavy non-critical workloads considering the hidden costs of Serverless.
Drop your comments, feedback, or suggestions below — or connect with me directly on Twitter @mlabouardy.